Why Deep Learning is not Artificial General Intelligence (AGI)
With the development in the field of deep learning, it has become a frontier in solving multiple challenging problems in computer vision, games, self-driving cars and many more. Deep learning has even achieved superhuman performance in some tasks, but still, it lacks some fundamental features which are required for a truly intelligent system. In this article, we are going to discuss some of these features and how they stop us from achieving artificial general intelligence (AGI).
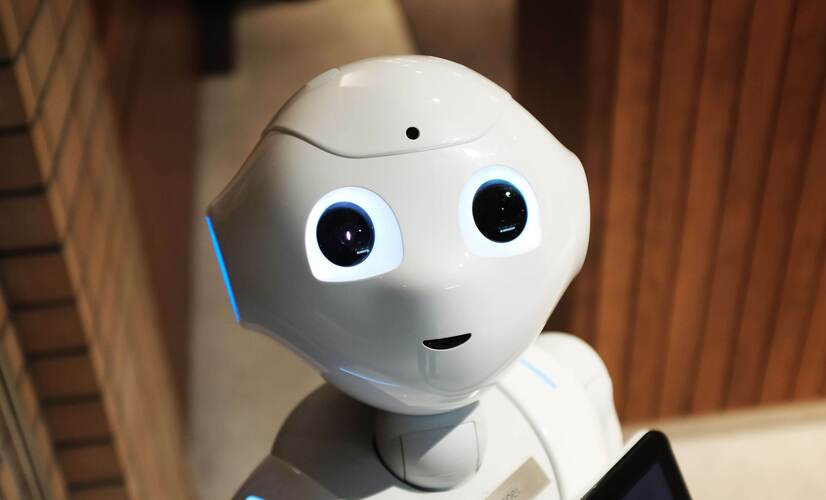
What is AGI?
Artificial General Intelligence (AGI) can be defined as a system or an agent capable of learning and solving tasks like a regular human being but with better speed and efficiency. AGI refers to the system that can simulate all the features of the human brain, including continual learning and unlearning, thinking, reasoning and decision-making.
In general, AGI can help humans solve tasks which are difficult for a human, as it has the human-like intelligence and speed of a computer.
Limitations of current Deep Learning
The current deep learning has some limitations due to which it cannot help in achieving Artificial General Intelligence (AGI) as we now know. Some of these limitations are as follows:
- Requirement of a large dataset
- Catastrophic forgetting
- No out-of-box thinking
- Lack of reasoning
- Vulnerable to different attacks
Requirement of Large Dataset
To get good performance, most deep-learning algorithms require a large set of training data, which are typically tens of thousands of samples. To gather such a large set of data samples and then labelling them accurately is quite expensive. As labelling consumes a lot of time and requires domain expertise which is hard to find in the case of complex domains such as medical imaging, satellite data and more.
As a human, we don’t need thousands of samples to learn a concept or an object. A few samples are enough to understand the concept and it gets improved over time. An AGI system needs to achieve human-like learning, i.e., it should require a few samples instead of thousands of samples.
Catastrophic forgetting
Most deep neural networks are built to accomplish a single task or problem. When these networks are given a new task to learn, they forget the information learned from the previous task. This phenomenon is known as catastrophic forgetting.
In simple terms, we can say that catastrophic forgetting prevents the deep neural networks from learning tasks or information in a continual fashion like a human. Due to this, we either need to learn all the information at once or build separate models. Both of these would make the scenario complex as the number of tasks grew.
To progress towards AGI, catastrophic forgetting needs to be solved so that the network has the ability to learn multiple tasks continually.
No Out-of-Box Thinking
Deep neural networks are trained for specific tasks due to which their predictions or outcomes are limited. These trained neural networks are not capable of adapting to new information and often fail to think out of the box. While humans use a lot of out-of-box thinking, which makes them effective in problem-solving.
These neural networks often fail to deliver correct results when the distribution of the input changes during testing and do not generalize well to new input (out-of-distribution).
Lack of Reasoning and Explainability
One of the main drawbacks of these deep neural networks is their inability to explain the reason for their decision. Due to this deep neural networks are also referred to as a black-box. To progress towards an AGI, this problem needs to be solved. Decisions without proper reasoning or explainability do not make sense, especially to humans.
While solving complex tasks, humans tend to come to conclusions with proper reason and explanation. Humans are capable of explaining their entire of reaching a decision.
Vulnerable to Different Attacks
The current version of deep neural networks is vulnerable to various adversarial attacks that may result in a loss of network performance drastically. A slight change in the image pixels or adding some noise changes the probabilities of the image classifier. Imagine a self-driving car being fooled because the camera has been obscured may result in a much worse driving experience or even a crash. An AGI system must have a built-in defence mechanism for such adversarial attacks.
Conclusion
Deep learning has proven its effectiveness in solving some of the most challenging problems, but there is still much room for improvement. Deep learning is still far from achieving Artificial General Intelligence (AGI) status. In this article, I have presented five limitations of current deep-learning techniques that I believe should be addressed in the coming years. Overcoming these limitations will enable us to build better neural networks which would further help us in building AGI.
For more, follow me on:
Hi Nikhil, Thanks for your dedications and explored very well. it is great helps to me. by Palani
ImportError: cannot import name 'load_data' from 'data' (C:\Users\Aravinda\anaconda3\lib\site-packages\data\__init__.py)
sir can you share a code of using squeeze and excitation network on custom CNN for a classification
Hey Nikhil, Very nice, to-the-point article on transfer learning. For a small size of dataset, an image augmentation along with…
Truly good blog short article and also valuable.